Ampere Goes Quantum: Get Your Qubits in the Cloud
by Dr. Ian Cutress on February 16, 2022 9:01 AM EST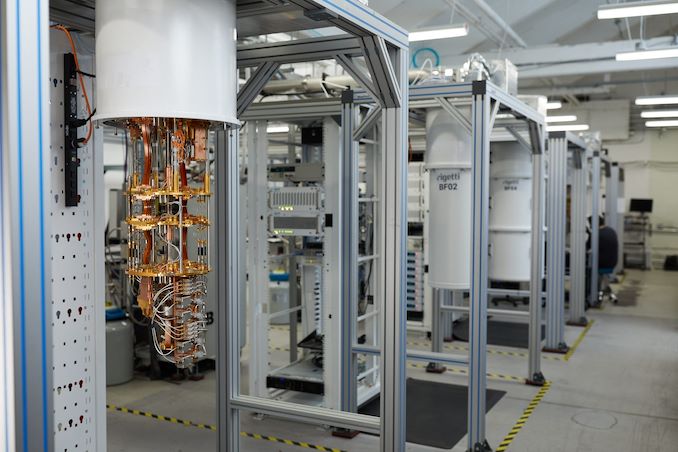
When we talk about quantum computing, there is always the focus on what the ‘quantum’ part of the solution is. Alongside those qubits is often a set of control circuitry, and classical computing power to help make sense of what the quantum bit does – in this instance, classical computing is our typical day-to-day x86 or Arm or others with ones and zeros, rather than the wave functions of quantum computing. Of course, the drive for working quantum computers has been a tough slog, and to be honest, I’m not 100% convinced it’s going to happen, but that doesn’t mean that companies in the industry aren’t working together for a solution. In this instance, we recently spoke with a quantum computing company called Rigetti, who are working with Ampere Computing who make Arm-based cloud processors called Altra, who are planning to introduce a hybrid quantum/classical solution for the cloud in 2023.
It’s All About the Qubits
The striking thing about quantum computing has always been the extravagant hardware required – a ‘golden steampunk chandelier’ of tubes and cables all required to bring the temperature of the hardware down hundredths of a degree above absolute zero. This minimizes thermal effects on the elements of a quantum computer, known as the qubit. Depending on the type of qubit involved, those cables can carry microwave signals, and how the chandelier is constructed often determines how many qubits are involved.
Qubits are the quantum computational power, and the more you have (in theory) the more exponentially more computing power there is on tap. However, because quantum computing doesn’t deal in absolutes, sometimes those qubits are used for resiliency, which is needed at such extreme environments. You’ll find that quantum computers list an ‘effective’ number of qubits equivalent to the computational power, rather than the actual physical number present. Beyond that, there are different types of Qubits.
Transmon qubits rely on superconducting electron pairs being controlled inside a three-dimensional cavity. A spin qubit controls individual electron spins with magnetic fields. Most companies use Transmon qubits (Google, IBM, Rigetti), whereas Intel dropped its Transmon development in favour of spin qubits. Exactly how many qubits a system needs to do ‘useful’ work is a hot topic in the literature, although Google claims it has performed computation impossible on classical computing with only 53 physical Transmon qubits – again, another hot topic for debate.
The ultimate goal of quantum computing is to enable computing resources that can solve classical problems whose compute requirements are impossible within reasonable time frames. The typical example is Shor’s Algorithm, to find prime factors of number (essentially solving the underlying basis for cryptography that should take millions of years) in seconds. Another example is solving a typically quantum-like system, such as chemistry and biochemical interactions. Also optimization, going beyond typical ‘traveling salesman’ into machine learning – the idea is that quantum computing can assist training or inference to check all possible answers, simultaneously.
Quantum computing has always been seen as a future horizon of where high-performance should go. However, it is one of those elements that always seems 10-20 years away. In the early 2000s it was seen as 10-20 years away, and the same is true today. However there are now more startups and funded ventures willing to put in more research to get these systems up and running. One of those is Rigetti, and today is an announcement of a collaboration with Ampere Computing.
Put The Quantum In The Cloud, Ampere Plus Rigetti
For the last few years, there has been a focus in putting high-performance computational resources within reach of everyone. The offering of cloud computing, web services, and 1000s of processors at your fingertips has never been more real, or been more easy. With enough money in your bucket, the cloud providers make it easy to spin up resources for storage, networking, services, or compute. Cloud computing like this is designed to scale as and when you need it. Rigetti wants to do the same with quantum computing.
Rigetti Computing, founded in 2013, is a series C funded quantum computing startup with a public $200m investment to date. Late last year, it announced the start of its new scalable quantum computing infrastructure – with a chip containing 40 transmon-style qubits, multiple chips can be embedded onto a single package for a single quantum computing chandelier. The goal of these designs is to accelerate machine learning, both for quantum compute and classical compute, and as a result, they’re partnering with Ampere Computing which makes the Altra Max Arm-based CPUs.
The goal of the partnership is to provide a cloud-native solution combining both classical and quantum computing. Spinning up an instance would include some qubits and some cores, allowing customers to use standard machine learning APIs that would be naturally split across the two types of hardware. In this heterogeneous combination, the goal is to take advantage of the quantum system to do what it does best, and then leverage the traditional compute resources with the Altra Max CPUs for machine learning scale out.
Rigetti says that its solution will scale to hundreds of qubits, while Ampere resources can scale as naturally as most compute can. Rigetti chose Ampere as a partner in this instance because of what the company can provide – Ampere always states that its processors are cloud-native, or built for the cloud, and that its 128-core chip can provide 1024 cores in a traditional 2U server with Arm Neoverse N1 performance.
At this point of the partnership, Rigetti and Ampere are at work developing a combination system up and running. Right now, the Ampere CPUs are to be part of the coupled performance resource, although Rigetti says that there could be a time where Ampere’s hardware might replace the FPGAs in the control units of the quantum system itself. The partnership aims to start working on a proof of concept, creating a local-to-Rigetti example of a cloud-native hybrid quantum/classical infrastructure, and creating a software stack optimized for machine learning. Rigetti says that it is already working with customers interested in the co-design to give itself targets for software optimizations.
The timeline for the rollout is still early, with a proof-of-concept planned over the next few months, then deployment with tier 1 cloud partners through 2023. The idea is to initially work with key customers to help optimize their workflows to combine with the hardware. Then it’s simply a case of scale out – more qubits for quantum, more CPUs for classical. Ampere is set to launch Siryn this year, its own custom Arm core built on next generation process node technology, and we were told that the scope is to bring in future Ampere generations as they are developed.
Rigetti says that it has made strides in enabling transmon qubits viable at scale. Intel dropped its transmon qubit program because it didn’t think it could scale, but also because they could create spin qubits fairly easily (however, control is a different part of that story). Rigetti plans to scale to the hundreds of qubits, allowing cloud customers to take a chunk of however many qubits they need at the time. One issue I brought up with them is synchronicity, and it sounds like they have a system that, in a traditional sense, can be asynchronous to scale. Rigetti believes there are elements to machine learning, both training and inference, that will scale with qubit count in this way.
Is Quantum Computing still a distant hope? The promise here is a hybrid product, with quantum and classical resources, for cloud customers in 2023. I fully expect that to be a viable use case. However, as is always the question with quantum computing – what problem is it solving, and is it better than classical?
24 Comments
View All Comments
GeoffreyA - Thursday, February 17, 2022 - link
According to physicist Paul Davies, owing to the fact that the complexity of an entangled state grows exponentially with increasing qubits, a very large qubit computer, such as a 400-component one if I understood him rightly, would come into conflict with a possible information bound in the universe. He reasons that if the universe were finite in resources, a maximum of 10^122 classical bits of information could be processed or contained in any causal region of the universe; and that scaling qubits beyond 400 or 500 would require more information than could fit in that bound. (I think it's related to the Bekenstein bound, which limits how much information can be stored in a region of space.) In short, if his arguments are right, the universe might have a physical limit that precludes practical quantum computing. Already, solving the issues of many-qubit decoherence seems to smack of this.https://arxiv.org/ftp/quant-ph/papers/0703/0703041...
Also, I remember reading that IBM disputed Google's claim. Apparently, some changes to the classical version could, or did, change it from hundreds of years, or something along those lines, to a few days. I quote the latter from memory, so apologies if I got it wrong.
mode_13h - Monday, February 21, 2022 - link
Thanks for that!It's funny how most of the discussion of this article seems focused on the Ampere piece, entirely ignoring the QC and machine learning aspects that set it apart from the standard fare.
GeoffreyA - Wednesday, February 23, 2022 - link
Yes, there could've been some fantastic discussion here on the quantum side of computing, which is still so difficult to grasp, much like its parent theory was for decades.mode_13h - Monday, February 21, 2022 - link
> into machine learning – the idea is that quantum computing can> assist training or inference to check all possible answers, simultaneously.
Not inference, I think, but training. Inference is much cheaper than training. However, the real allure of applying QC to training is the possibility of finding the globally optimal set of weights, whereas classical training methods can only converge on somewhat locally-optimal configurations. This should enable greater accuracy per node, which can enable smaller networks requiring less power and memory to inference.